💡 Takeaway:
Peer-implied mispricing from accounting statements is a powerful predictor of future returns—without using any forward-looking or discretionary inputs.
Key Idea: What Is This Paper About?
Bartram and Grinblatt develop a statistician’s approach to valuation: estimate fair value using cross-sectional regressions of market cap on all accounting variables—without forecasting cash flows or selecting models. The mispricing signal is the percent deviation of a firm’s market value from its peer-implied value. This simple approach leads to profitable convergence trades—buying underpriced and shorting overpriced firms—earning significant alpha.
Economic Rationale: Why Should This Work?
📌 Relevant Economic Theories and Justifications:
- Law of One Price: Firms with identical fundamentals should trade at similar values.
- Convergence to Fair Value: Market prices tend to correct over time toward fundamental anchors.
- Information Diffusion: Investors underreact to complex or dispersed accounting information.
- Statistical Regularity: By pooling information from peers, the model exploits common valuation norms.
📌 Why It Matters:
This method avoids overfitting, data snooping, and discretionary model choices—delivering robust, statistically significant alpha from first principles.
Data, Model, and Strategy Implementation
Data Used
- Period: March 1987 – December 2012
- Universe: US nonfinancial firms on NYSE, Amex, Nasdaq
- Inputs: 28 balance sheet, income, and cash flow items from Compustat Point-in-Time (PIT)
- Exclusions: Financial firms, share price < $5
Model / Methodology
- Peer-Implied Fair Value:
- Cross-sectional regression: Market cap ~ 28 accounting variables
- Use OLS or Theil–Sen median estimator
- We define the model's mispricing measure for stock j at time t as the percentage deviation of the predicted price from the fundamental value. Specifically, it is the difference between the machine learning model's forecasted price and the stock’s estimated fundamental value, scaled by the fundamental value itself.
- Quintile Sorting: Rank firms monthly by mispricing; Q1 = most overpriced, Q5 = most underpriced
- Control Variables (for robustness): Size, B/M, momentum, accruals, SUE, gross profitability, earnings yield
Trading Strategy (Mispricing Signal)
- Signal:
- Long Q5 (most underpriced), short Q1 (most overpriced)
- Use either OLS or robust Theil–Sen predicted values
- Rebalance: Monthly
- Enhancements:
- Strongest in small caps
- Signal decay observed—returns converge over 3 years
- Works equally well out-of-sample, with no reliance on future data
Key Table or Figure from the Paper
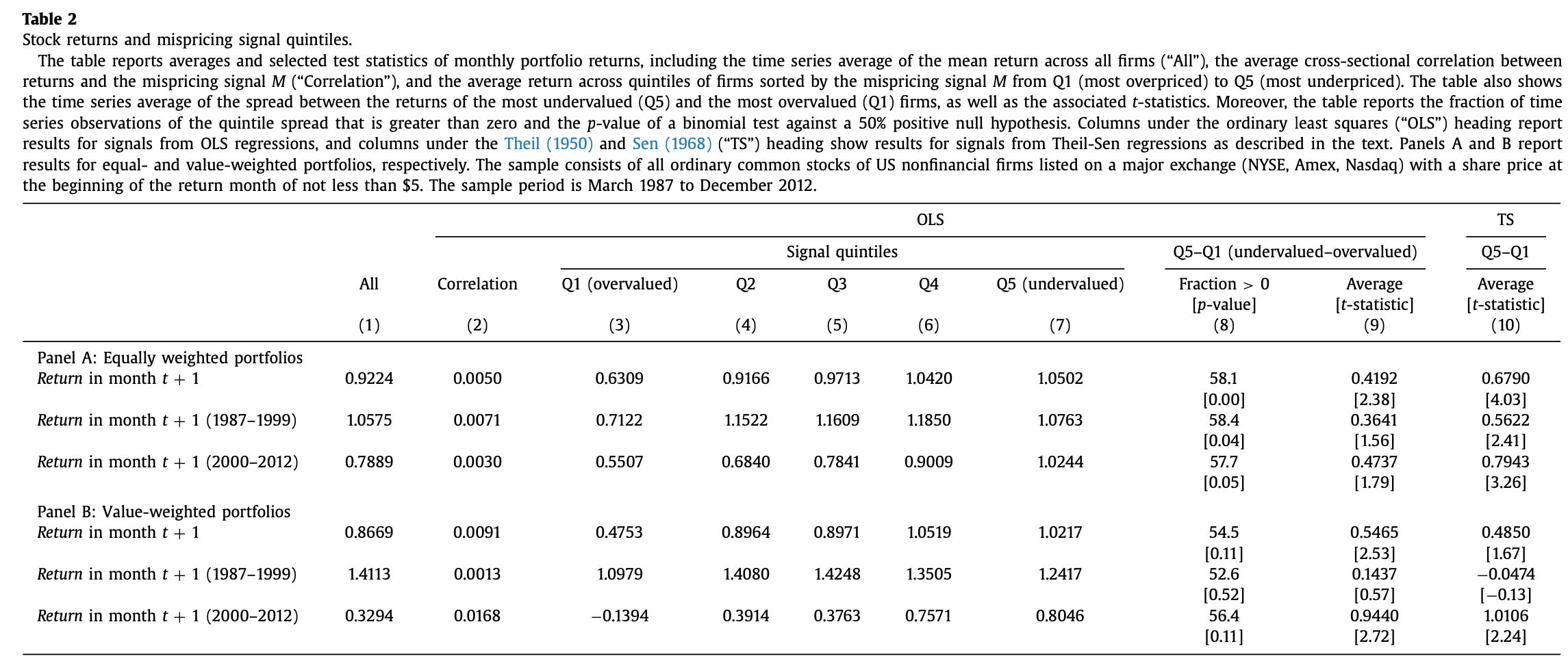
📊 Reference: [Table 2] – Returns by Mispricing Quintiles
📌 Explanation:
- Equally-weighted Q5–Q1 spread: 0.42%–0.68% per month, depending on estimation method
- Value-weighted Q5–Q1 spread: ~0.55% per month
- Returns are monotonic in mispricing; results persist across subperiods
- Signals remain significant after controlling for 28 other anomalies
Final Thought
💡 You don’t need DCF models—just let the data reveal what’s mispriced. 📊🚀
Paper Details (For Further Reading)
- Title: Agnostic Fundamental Analysis Works
- Authors: Söhnke M. Bartram, Mark Grinblatt
- Publication Year: 2018
- Journal/Source: Journal of Financial Economics
- Link: https://doi.org/10.1016/j.jfineco.2016.11.008