Takeaway:
Summaries generated by GPT-3.5 are shorter yet significantly more informative than full MD&As and earnings calls. Summary sentiment explains market reactions better than the full text. Bloat in disclosures correlates with lower price efficiency and higher info asymmetry.
Key Idea: What Is This Paper About?
The paper shows that GPT-3.5 can distill long and bloated MD&A and earnings call transcripts into concise, focused summaries. When you measure sentiment from these summaries, it predicts short-term stock returns better than using the raw documents. The authors also create a “Bloat” score to quantify how much fluff a disclosure contains — and show that high bloat correlates with worse market efficiency.
Economic Rationale: Why Should This Work?
Investors have limited attention. ChatGPT acts as a cognitive assistant that summarizes and clarifies what matters most. It amplifies the tone of the underlying disclosure, removing filler and revealing clearer sentiment that markets respond to.
Relevant Economic Theories and Justifications:
- Rational Inattention (Sims, 2003): Investors can’t process everything. GPT reduces noise so they can act faster.
- Obfuscation Hypothesis (Li, 2008): Managers bury bad news in long, complex language. GPT cuts through it.
- Limits to Arbitrage & Market Frictions (Leuz & Verrecchia, 2000): Bloat increases frictions and slows price discovery. AI summaries restore clarity.
Why It Matters:
Sentiment from raw filings is weak. Sentiment from GPT summaries is sharp and predictive — it’s a clean alpha signal.
Data, Model, and Strategy Implementation
Data Used
- Data Sources:
- MD&A from 10-Ks (EDGAR)
- Earnings Calls (CapitalIQ)
- CRSP, TAQ, Compustat, I/B/E/S
- Time Period: 2009–2020 (main sample), 2022 (out-of-sample test)
- Asset Universe: US-listed, non-financial firms
Model / Methodology
-
Type of Model: GPT-3.5 Turbo
-
Approach: Zero-shot summarization of each filing using OpenAI API
-
Prompt Used:
“Summarize the following text using only the information included in the text.
Do not restrict the length of the summary.
Do not use information from outside the document.” -
Sentiment Measurement:
Loughran-McDonald sentiment dictionary applied to the summary (not the original text) -
Bloat Measure:
Bloat = (Original Length − Summary Length) / Original Length
Trading Strategy (Based on Summary Sentiment)
Main Idea:
Use GPT summary sentiment as a long-short signal around earnings and 10-Ks.
-
Signal Generation:
- Use GPT-3.5 to summarize MD&A or call transcript
- Apply Loughran-McDonald dictionary to compute summary sentiment score
- Use the sentiment score as your alpha signal
-
Portfolio Construction:
- Long top 20–30% most positive sentiment
- Short bottom 20–30% (or avoid if long-only)
- Equal-weighted or volatility-adjusted
-
Rebalancing Frequency:
- Annually for 10-K MD&A-based signals
- Quarterly for earnings call-based signals
-
Enhancement Ideas:
- Overlay with traditional earnings surprise or momentum
- Use Bloat score to filter: High Bloat + Negative Sentiment = strong short
Key Table or Figure from the Paper
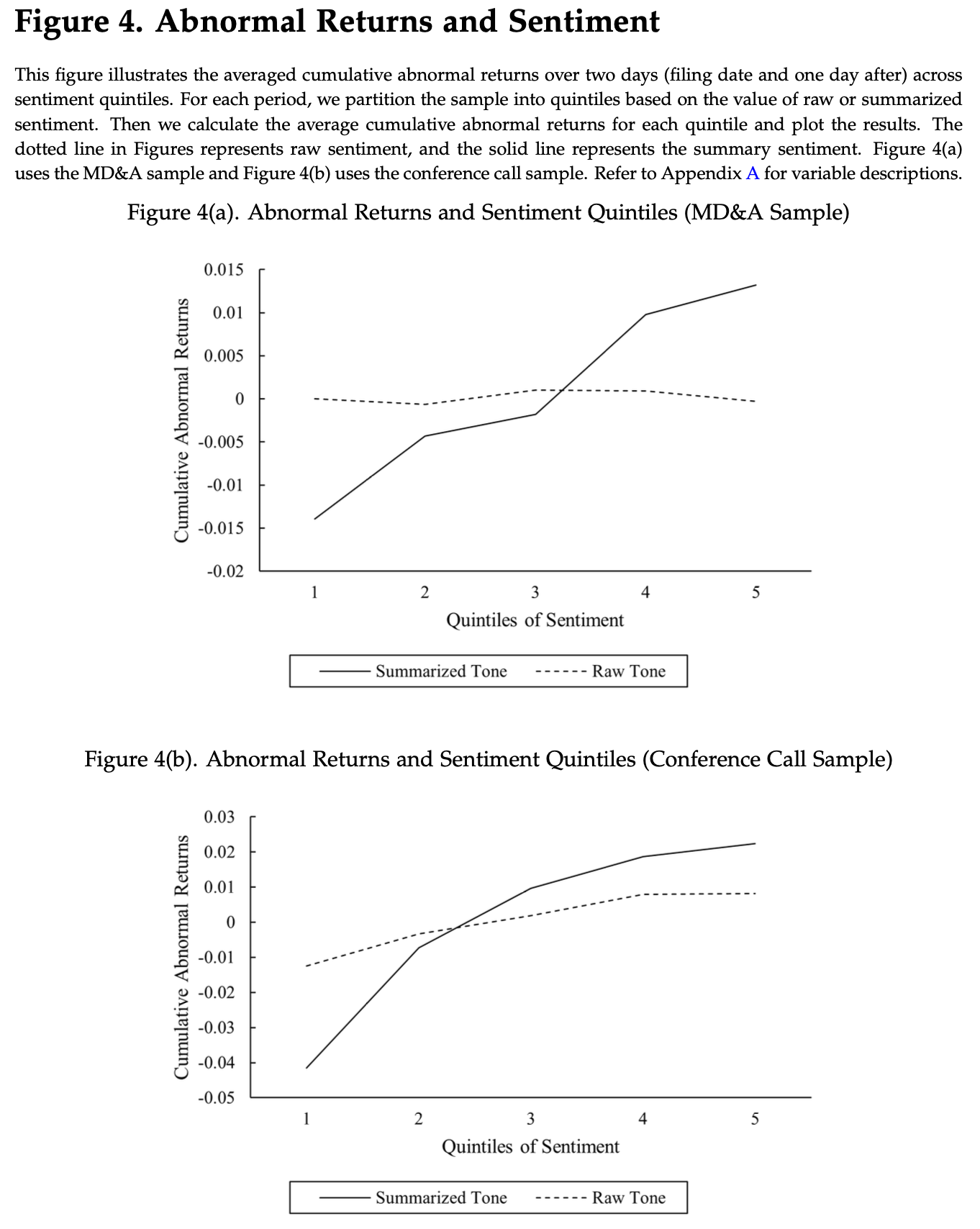
Reference: [Figure 4 – Abnormal Returns by Sentiment Quintile]
Explanation:
- Stocks sorted by sentiment quintiles
- Raw sentiment: flat, noisy
- Summary sentiment: clear monotonic return pattern
- Confirms GPT summaries extract investable sentiment that traditional methods miss
💡 Summary Sentiment vs. Bloat: What’s the Difference?
Metric | What It Tells You | Use Case |
---|---|---|
Sentiment (GPT Summary) | Directional signal → stock goes up/down | Trade it (alpha) |
Bloat | Measures noise or obfuscation | Filter it (diagnostic) |
Use Bloat to understand why the summary sentiment works — especially in noisy, hard-to-read disclosures.
Final Thought
💡 Generative AI isn’t just summarizing text—it’s summarizing alpha. 📈
Paper Details (For Further Reading)
- Title: Bloated Disclosures: Can ChatGPT Help Investors Process Information?
- Authors: Alex G. Kim, Maximilian Muhn, Valeri V. Nikolaev
- Publication Year: 2024
- Journal/Source: Working Paper
- Link: https://arxiv.org/abs/2306.10224