Takeaway:
AI democratization enables retail investors to act on information once only usable by sophisticated traders. ChatGPT-based sentiment forecasts stock returns, improves retail trading, and narrows the information gap.
Key Idea: What Is This Paper About?
This paper examines how ChatGPT’s AI-sentiment extracted from long-form earnings calls improves return predictability and helps retail investors compete with short sellers. Before ChatGPT’s launch, only informed traders aligned with AI-sentiment. Post-launch, retail traders shift their behavior to match AI insights, increasing alignment by 23x.
Economic Rationale: Why Should This Work?
AI-sentiment captures long-text context, filters hype, and identifies when positive language masks bad news. Retail investors can now access these insights without advanced tools.
Relevant Economic Theories and Justifications:
- Information Frictions: AI reduces the gap between information-rich and poor investors
- Limits to Arbitrage: Short sellers previously used this edge; now retail investors can too
- Democratization Effects: Like Reg FD but with processing power, not just access
Why It Matters:
AI shifts the informational power balance in markets. Widespread, low-cost access to tools like ChatGPT allows ordinary investors to generate insights once exclusive to institutions.
How to Do It: Data, Model, and Strategy Implementation
Data Used
- Data Sources: S&P Capital IQ, TAQ, CRSP, Compustat
- Time Period: 2005–2023 (focus: 2010–2023)
- Asset Universe: US common stocks on NYSE, NASDAQ, AMEX
Model / Methodology
- Type of Model: GPT-3.5-turbo-16k + Regression + DiD framework
- Key Features:
- Full earnings call transcripts (avg. 7,000 words) fed to ChatGPT
- Returns predicted using AI-sentiment (scale -10 to +10)
- Compared to HD-sentiment (LM dictionary) and FinBERT
- Used event-time regressions and portfolio sorts
Prompt:
"Forget all your previous instructions. You are a stock market trader with experience in both fundamental analysis and technical analysis. Knowledge cutoff: {date}. Can you define the concept of conference call transcript? I will give you a transcript. Describe its sentiment on a scale from -10 (very negative) to 10 (very positive)."
Trading Strategy
-
Signal Generation:
- Monthly AI-sentiment scores from latest earnings calls
- Double-sorted with HD-sentiment or SUE for robustness
-
Portfolio Construction:
- Long top decile, short bottom decile of AI-sentiment scores
- Equal- and value-weighted (capped at 80%)
- Alpha persists for 6 months post-call
-
Rebalancing Frequency: Monthly
Key Table or Figure from the Paper
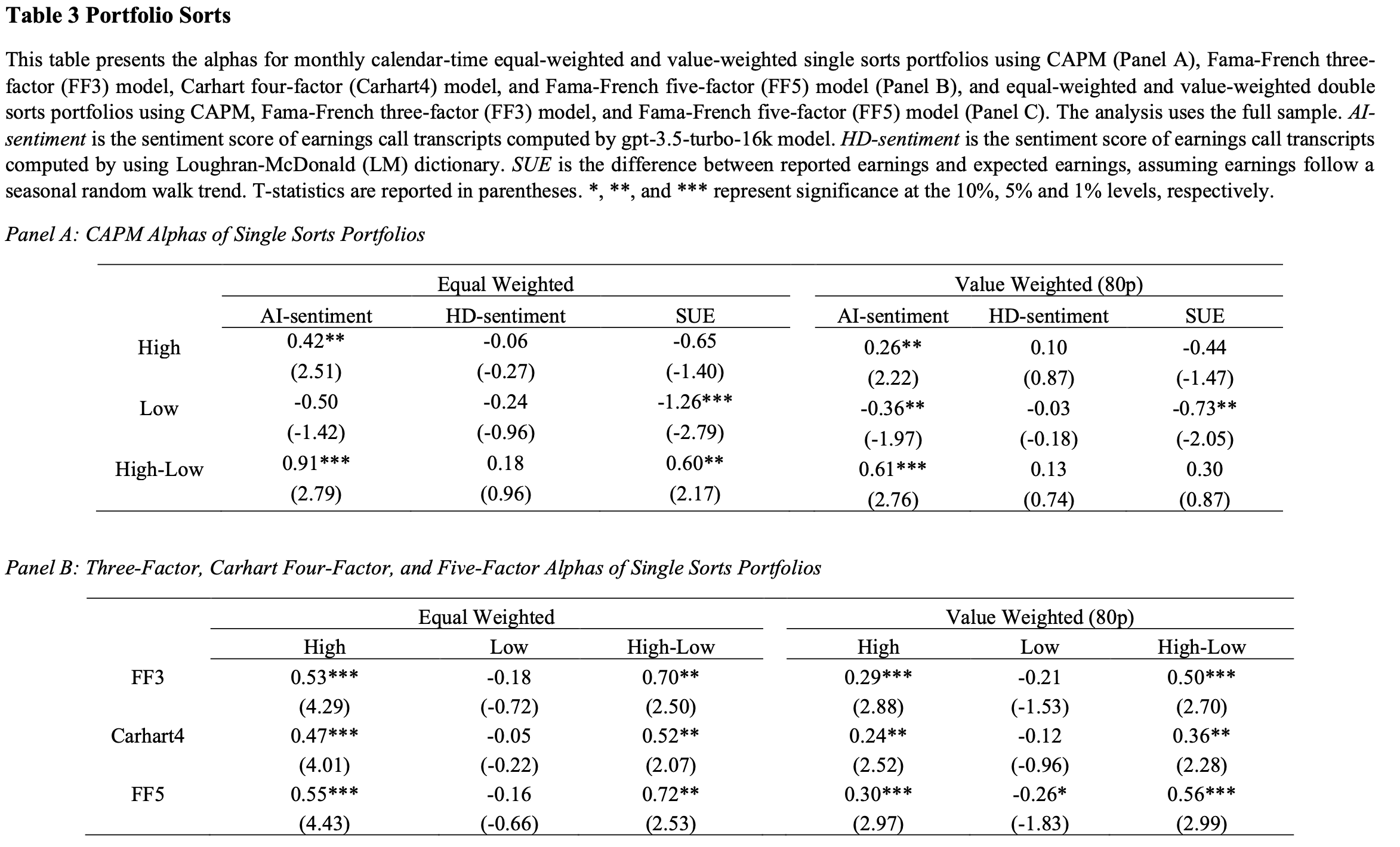
Reference: [Table 3 – Portfolio Alphas from AI-Sentiment]
Explanation:
- Equal-weighted long-short: 0.91% monthly CAPM alpha
- Value-weighted: 0.61% monthly CAPM alpha
- Fama-French 3/4/5-factor alpha: 0.36–0.72% per month
- No alpha from HD-sentiment or FinBERT-based strategies
Final Thought
💡 ChatGPT empowers the crowd—retail traders now compete on the same insights as Wall Street. 💥📊
Paper Details (For Further Reading)
- Title: AI (ChatGPT) Democratization, Return Predictability, and Trading Inequality
- Authors: Anne Chang, Xi Dong, Xiumin Martin, Changyun Zhou
- Publication Year: 2024
- Journal/Source: SSRN Preprint
- Link: https://ssrn.com/abstract=4543999