đĄ Takeaway:
ChatGPT captures commodity sentiment better than BERT, BoW, or macroeconomic variablesâoffering robust, predictive signals for commodity futures returns.
Key Idea: What Is This Paper About?
The paper constructs a Commodity News Ratio Index (CNRI) by analyzing 2.5M news articles using ChatGPT. Each article is scored based on whether ChatGPT believes it signals a price increase or decrease. These sentiment signals are aggregated into a commodity-level CNRI and distilled using Partial Least Squares (PLS) to forecast commodity futures index excess returns.
Economic Rationale: Why Should This Work?
đ Relevant Economic Theories and Justifications:
- Investor Sentiment: Media sentiment influences commodity markets, especially when driven by global narratives.
- LLMs Extract Informational Signals: ChatGPT outperforms classical NLP (BoW, BERT) by capturing deeper semantics and context.
- Slow News Diffusion: Commodities are less covered in financial media, allowing sentiment to diffuse slowly into prices.
- Predictive Link to Macro: CNRI also predicts GDP, IPI, CFNAI, and PPIâsuggesting macroeconomic underpinnings.
đ Why It Matters:
This approach provides forward-looking, interpretable, and systematic sentiment-driven signals using LLMsâextending NLP-based forecasting to commodities.
How to Do It: Data, Model, and Strategy Implementation
Data Used
- Text Corpus: 2.6M articles (1946â2022) from 9 major newspapers
- Commodities: 18 (e.g., crude oil, gold, soybeans, zinc)
- Returns: Monthly commodity futures index excess returns (Levine et al. 2018)
Model / Methodology
- Step 1: Use ChatGPT to classify articles as âGOING UPâ, âGOING DOWNâ, or âUNKNOWNâ
- Step 2: Compute a Commodity News Ratio (CNR) = (Good â Bad) / Total articles (past 3 months)
- Step 3: Use PLS to form the ChatGPT-based CNRI from 18 CNRs
- Step 4: Forecast commodity index returns using CNRI
- Comparison Models: BERT-based CNRI, BoW-based CNRI, macroeconomic indicators, business cycle controls
Trading Strategy (Investor Application)
- Signal: Use CNRI to forecast next 1â12 months of commodity index returns
- Portfolio Construction:
- Allocate between commodity futures and T-bills
- Use CNRI forecasts in mean-variance optimization
- Volatility estimated using 12-month rolling GSCI standard deviation
- Performance:
- Highest Sharpe: 0.407 (3-month horizon)
- Highest CER: 5.86% (1-month horizon)
- Robust across macro states (recessions, backwardation, inflation down)
Key Table or Figure from the Paper
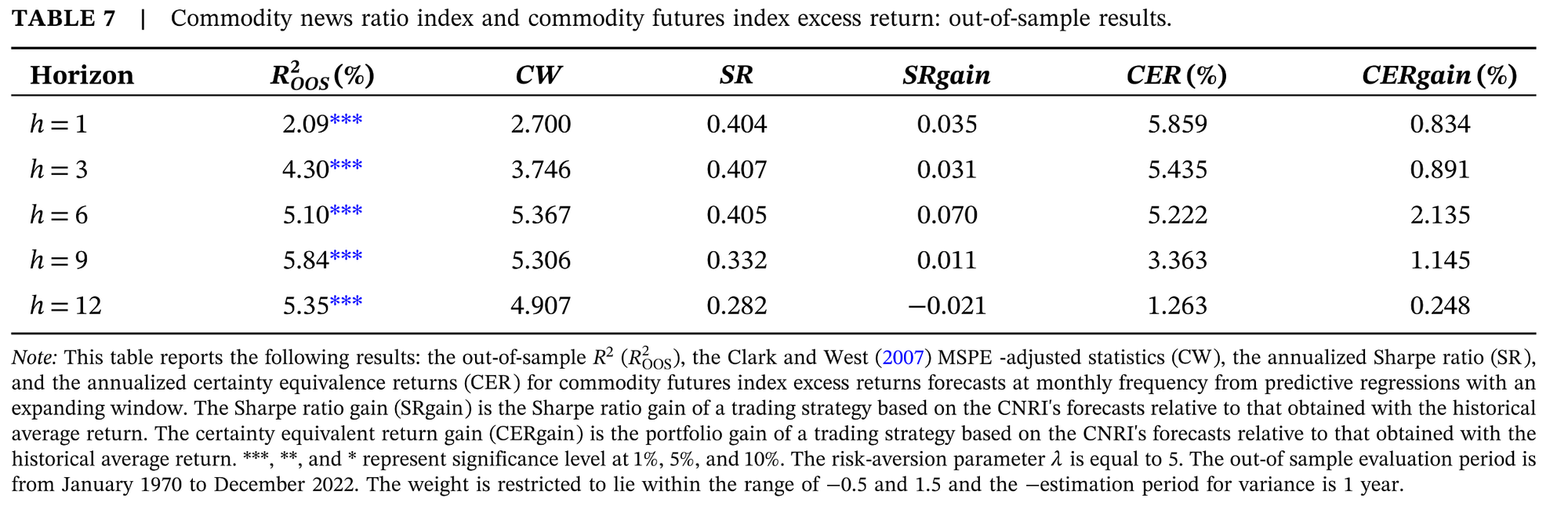
đ Reference: [Table 7] â Out-of-Sample Forecasting and Portfolio Performance
đ Explanation:
- Shows the CNRI achieves out-of-sample R² from 2.09% to 5.84%
- Sharpe Ratios exceed benchmark across all horizons
- CER gains peak at 2.1%â2.2% over 6â9 month horizons
- CNRI outperforms both economic indicators and BERT-based sentiment
Final Thought
đĄ ChatGPT decodes commodity headlines into portfolio alphaâNLP for futures trading is here. đ
Paper Details (For Further Reading)
- Title: ChatGPT and Commodity Return
- Authors: Shen Gao, Shijie Wang, Yuanzhi Wang, Qunzi Zhang
- Publication Year: 2025
- Journal/Source: Journal of Futures Markets
- Link: https://doi.org/10.1002/fut.22568