Takeaway:
ChatGPT’s investment score forecasts real investment and returns. High-score firms invest more and underperform—matching investment-based asset pricing logic.
Key Idea: What Is This Paper About?
The paper processes 74,586 earnings calls using ChatGPT-3.5 to extract forward-looking corporate investment sentiment. The resulting score predicts future capital expenditures for up to 9 quarters and forecasts negative abnormal stock returns—providing a novel, interpretable alpha signal.
Economic Rationale: Why Should This Work?
Earnings calls contain forward-looking management expectations that aren't always priced in. ChatGPT captures these signals better than traditional models or analysts.
Relevant Economic Theories and Justifications:
- q-Theory of Investment – Investment depends on expected returns and value; ChatGPT extracts info beyond Tobin's q.
- Behavioral Underreaction – Investors may underweight qualitative forward-looking signals.
- Investment-Based Asset Pricing – High investment predicts lower returns (Liu, Whited & Zhang 2009).
Why It Matters:
ChatGPT surfaces overlooked investment tone from public disclosures—turning text into predictive signals for asset managers and researchers.
How to Do It: Data, Model, and Strategy Implementation
Data Used (If Applicable)
- Data Sources:
- Earnings call transcripts (Seeking Alpha)
- CRSP / Compustat
- Duke CFO Survey
- Time Period: 2006–2020 (main sample), 2021–2022 (out-of-sample test)
- Asset Universe: US public firms with quarterly earnings calls
Model / Methodology (If Applicable)
- Type of Model: ChatGPT-3.5 Zero-Shot Classification + Panel Regression
- ChatGPT prompted with:
"The following text is an excerpt from a company’s earnings call transcripts.
You are a finance expert. Based on this text only, please answer the following question.
How does the firm plan to change its capital spending over the next year?
There are five choices: Increase substantially, increase, no change, decrease, and decrease substantially.
Please select one of the above five choices for each question and provide a one-sentence explanation of your choice.
The format should be: “choice - explanation.” If no relevant info is found, answer “no information is provided."
- Scoring System:
- Increase substantially → +1
- Increase → +0.5
- No change → 0
- Decrease → −0.5
- Decrease substantially → −1
- Average score across chunks = firm-quarter ChatGPT Investment Score
Trading Strategy (If Applicable)
Main Idea:
Firms with higher expected capex underperform → short them. Low-score firms (cutting investment) outperform → long them.
- Signal Generation: Rank firms by ChatGPT investment score post-earnings call
- Portfolio Construction:
- Long bottom decile (low score)
- Short top decile (high score)
- Rebalancing Frequency: Quarterly
- Enhancements:
- Combine with quality/momentum
- Filter for liquidity and earnings surprise
Key Table or Figure from the Paper
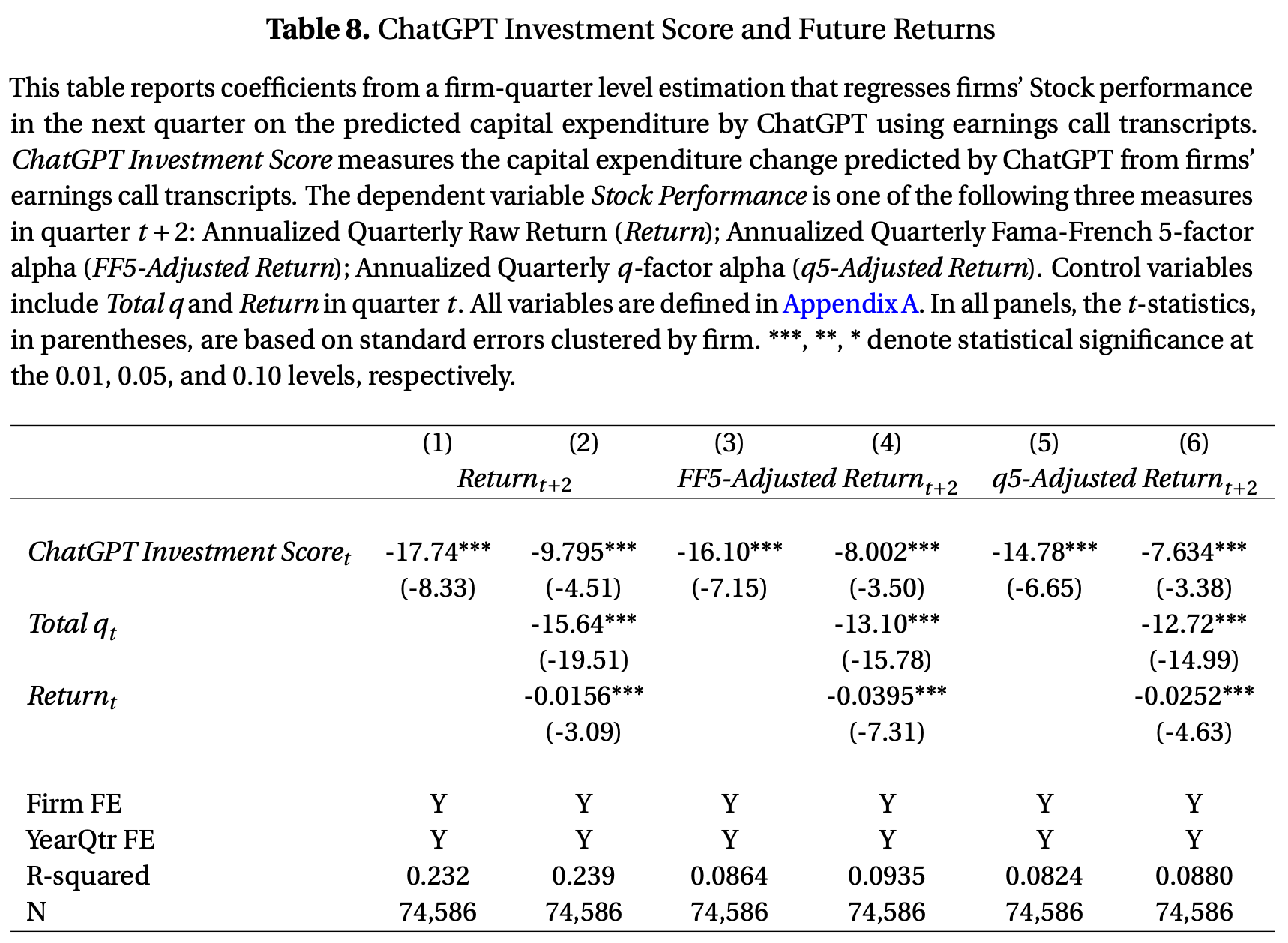
Reference: [Table 8 – ChatGPT Investment Score and Future Returns]
Explanation:
- A one-standard-deviation increase in the investment score →
- −1.80% raw return
- −1.47% FF5-adjusted return
- −1.40% q5-adjusted return
- Predictive effect lasts up to 9 quarters ahead
- Confirmed across factor models and controlling for other signals
Final Thought
💡 ChatGPT decodes capex tone in earnings calls—and sees mispricing the market misses. 🧠📉
Paper Details (For Further Reading)
- Title: ChatGPT and Corporate Policies
- Authors: Manish Jha, Jialin Qian, Michael Weber, Baozhong Yang
- Publication Year: 2024
- Journal/Source: NBER Working Paper No. 32161
- Link: https://www.nber.org/papers/w32161