Takeaway:
GPT-4 can classify Federal Reserve policy tone better than traditional NLP methods and help identify macroeconomic policy shocks—offering a new way to forecast monetary policy changes.
Key Idea: What Is This Paper About?
This paper evaluates how well GPT models, including ChatGPT and GPT-4, interpret Federal Reserve communications (a.k.a. “Fedspeak”). It shows that GPT models not only outperform other NLP tools (like BERT and dictionaries) in classifying policy tone, but can also replicate the narrative approach of Romer & Romer (1989) to identify monetary policy shocks—automating a task previously done by experts.
Economic Rationale: Why Should This Work?
Fed announcements are complex, nuanced, and crucial for asset pricing. GPT models excel at understanding language context, making them well-suited to interpret central bank tone and intent.
Relevant Economic Theories and Justifications:
- Central Bank Signaling Theory: Monetary policy guidance impacts expectations and asset prices.
- Narrative Monetary Policy Identification (Romer & Romer): Determining policy shocks requires reading through qualitative Fed transcripts—GPT-4 can now replicate this task.
- Information Frictions: GPT helps eliminate interpretation gaps across investors, improving informational efficiency.
Why It Matters:
Understanding Fed tone helps predict rate decisions and risk sentiment. GPT democratizes access to these signals—making macro-driven trading more scalable and accurate.
How to Do It: Data, Model, and Strategy Implementation
Data Used (If Applicable)
- Data Sources:
- FOMC announcements (2010–2020)
- Fed transcripts and meeting minutes (1946–2023)
- Time Period:
- Classification task: 2010–2020
- Narrative analysis: 1946–2023
- Asset Universe: Not applicable (Fed communications used to generate macro signals)
Model / Methodology (If Applicable)
- Type of Model: GPT-3, GPT-4, ChatGPT (zero-shot and fine-tuned)
- Key Features:
- Classification task: Label FOMC sentence as dovish/neutral/hawkish
- Narrative task: Determine if a transcript contains a monetary policy shock
- Human benchmark used: Research assistant named “Bryson”
Prompt for Classification Task:
"Imagine you are a research assistant working for the Fed. You have a degree in Economics.
Classify the sentence into: dovish, mostly dovish, neutral, mostly hawkish, hawkish."
Prompt for Narrative Policy Shock Detection (Romer & Romer style):
"As a monetary policy expert, determine if this text contains a policy shock:
• Was the economy at potential?
• Was there a policy move due to inflation?
• Were policymakers willing to accept output/unemployment pain?
Provide reasoning if yes/no."
Trading Strategy (Reframed from Findings)
While no strategy is explicitly tested, the findings can be translated into macro signal generation:
-
Signal Generation:
- Use ChatGPT to classify FOMC announcements and transcripts into dovish/hawkish
- Track tone changes or identify shock events
-
Portfolio Construction:
- Long bonds when GPT sentiment shifts dovish
- Short USD / long equities during easing surprises
- Use narrative-based shock detection to anticipate volatility or risk repricing
-
Rebalancing Frequency:
- Around each Fed meeting (8x per year)
- More frequent if incorporating GPT signal changes in real-time
Key Table or Figure from the Paper
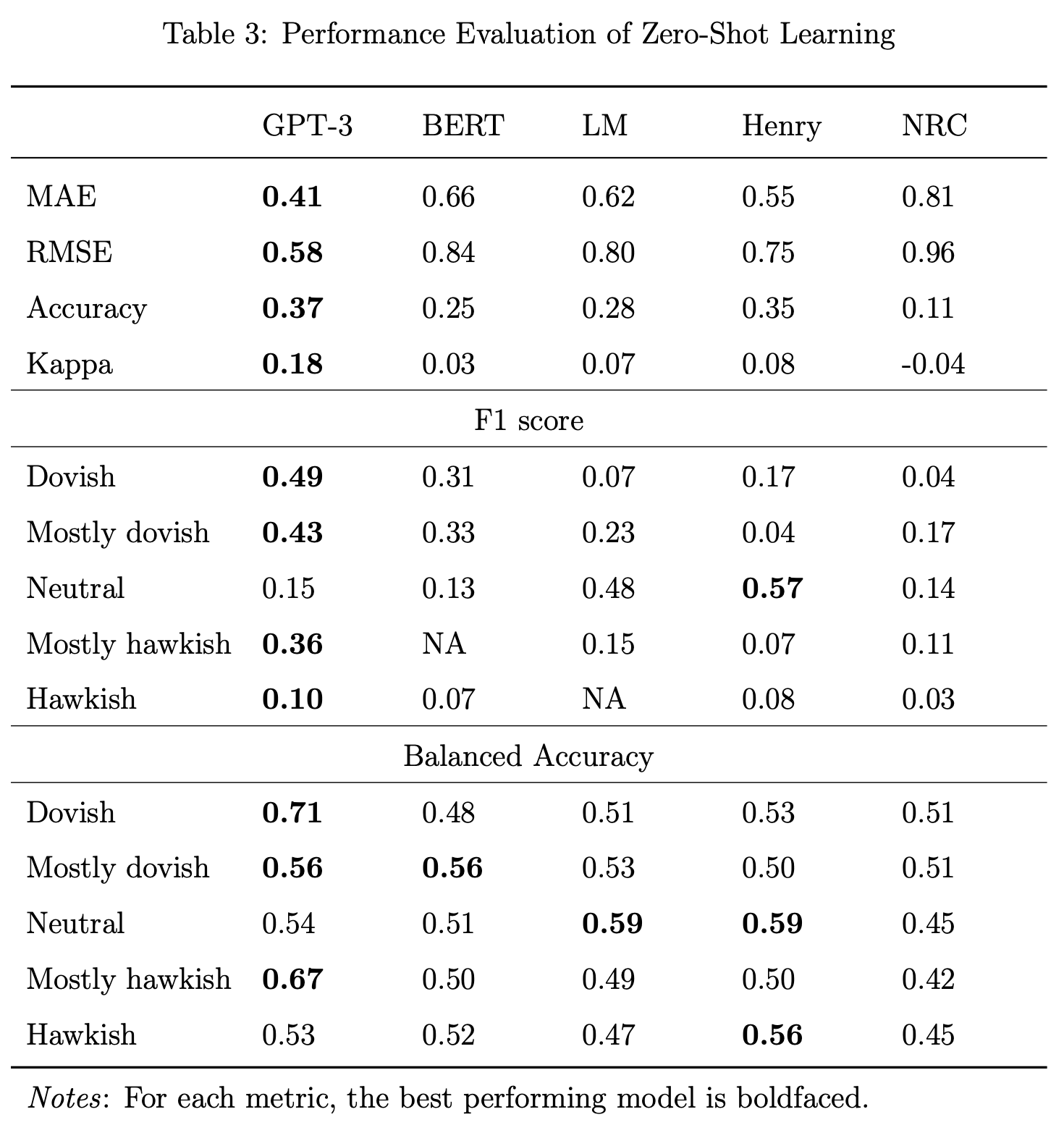
Reference: [Table 3 – Performance Evaluation of Zero-Shot Learning]
Explanation:
- GPT-3 outperforms all other models with 37% accuracy (vs. 25% for BERT)
- Fine-tuning improves this to 61% accuracy
- GPT also provides high-quality explanations for its predictions, aligning with human logic
Final Thought
💡 GPT-4 can read the Fed like an economist—and turn central bank language into actionable macro insights. 🧠📉
Paper Details (For Further Reading)
- Title: Can ChatGPT Decipher Fedspeak?
- Authors: Anne Lundgaard Hansen, Sophia Kazinnik
- Publication Year: 2023
- Journal/Source: SSRN Preprint
- Link: https://ssrn.com/abstract=4399406