đź’ˇ Takeaway:
ChatGPT-identified "good news" from WSJ headlines consistently predicts future stock market returns and significantly improves asset allocation outcomes.
Key Idea: What Is This Paper About?
This paper shows that ChatGPT can extract forward-looking signals from Wall Street Journal headlines that predict the US stock market's aggregate returns. Unlike other models (DeepSeek, BERT, word lists), ChatGPT’s sentiment classification captures economic fundamentals that investors underreact to—especially in the case of positive news.
Economic Rationale: Why Should This Work?
ChatGPT reveals "good news" signals overlooked by markets due to human bias, ambiguity aversion, and attention constraints.
📌 Relevant Economic Theories and Justifications:
- Ambiguity Aversion (Epstein & Schneider, 2008): Investors discount unclear good news.
- Asymmetric Reactions (Veronesi, 1999): Markets respond slower to good news in bad times.
- Limits to Information Processing (Zhang, 2006; Chan et al., 1996): Good news is often novel or complex.
- Inattention & Sentiment Bias (DellaVigna & Pollet, 2009): Negative news gets instant attention, while good news doesn’t.
📌 Why It Matters:
These behavioral frictions create a window where AI can outperform human investors by systematically capturing delayed reactions to good news.
How to Do It: Data, Model, and Strategy Implementation
Data Used
- Data Sources: Front-page headlines from the Wall Street Journal (Factiva)
- Time Period: Jan 1996 – Dec 2022
- Asset Universe: S&P 500 Index (Market portfolio)
Model / Methodology
- Type of Model: Large Language Model (ChatGPT-3.5)
- Prompt Design:
“Is this headline GOOD or BAD for U.S. stock prices?”
- Output Classification: GOING UP / GOING DOWN / UNKNOWN
- Signal Metric:
- NRG = % of monthly headlines classified as "good"
- NRB = % of monthly headlines classified as "bad"
Trading Strategy
-
Signal Generation: Use NRG (good news ratio) to forecast future returns
-
Portfolio Construction:
- Mean-variance optimized portfolio between market and risk-free asset
-
Rebalancing Frequency: Monthly
-
Constraints: No short-selling, max leverage 1.5x
-
Enhancements:
- Fine-tuning ChatGPT on financial headlines improves signal quality
- Combining with DeepSeek/BERT adds investor sentiment layer
Key Table or Figure from the Paper
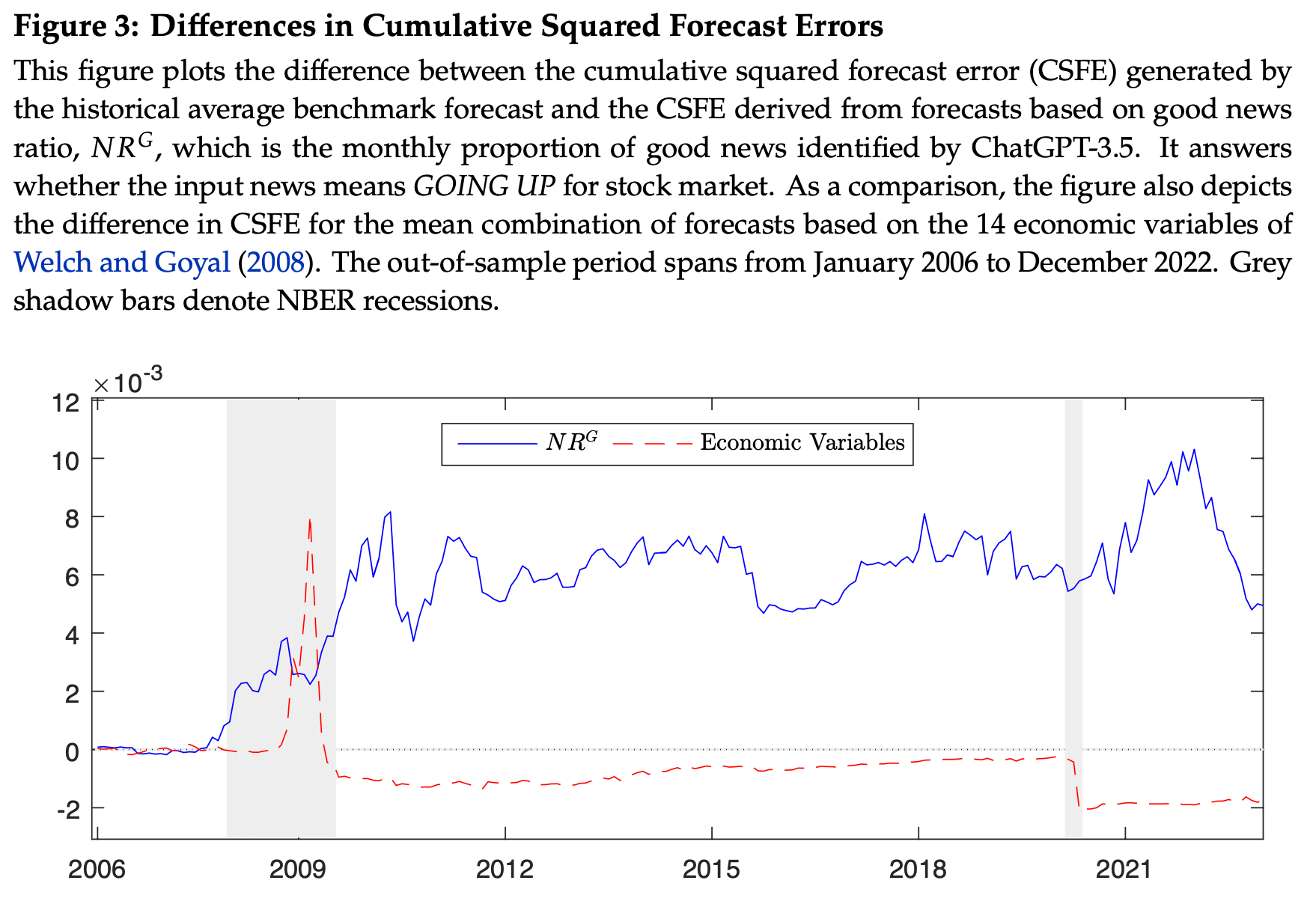
📌 Explanation:
- This figure compares the predictive performance of ChatGPT (NRG-based forecast) vs. economic variable combinations.
- The upward trend in the NRG curve indicates ChatGPT consistently beats the historical average in forecasting market returns.
- Especially strong performance around the 2008 crisis and COVID-19 periods.
Final Thought
💡 ChatGPT can spot good news the market ignores—and that's alpha. 🚀
Paper Details (For Further Reading)
- Title: ChatGPT and Deepseek: Can They Predict the Stock Market and Macroeconomy?
- Authors: Jian Chen, Guohao Tang, Guofu Zhou, Wu Zhu
- Publication Year: 2025
- Journal/Source: arXiv (Preprint)
- Link: https://arxiv.org/abs/2502.10008