Takeaway:
Generative AI adoption drives alpha among hedge funds, especially those with AI-skilled staff. Larger, active funds benefit most from analyzing firm-level info using ChatGPT.
Key Idea: What Is This Paper About?
The paper develops a novel measure (RAI — Reliance on AI Information ) to quantify hedge funds' reliance on ChatGPT-generated firm-level insights from earnings calls. Funds using this information more heavily earn significantly higher returns post-2022. AI adoption appears to enhance stock-level insights, but only for well-resourced, sophisticated firms.
Economic Rationale: Why Should This Work?
ChatGPT can process vast unstructured data and extract predictive firm-level signals, improving decision-making in security selection. Well-resourced hedge funds can better integrate these insights.
Relevant Economic Theories and Justifications:
- Grossman-Stiglitz (1980): Investors earn alpha by processing information more efficiently
- Information Advantage: Early adopters of new tech extract latent alpha
- AI-Human Complementarity: AI tools amplify performance when paired with expertise
Why It Matters:
This is the first large-scale evidence that generative AI materially improves fund performance—and highlights the growing disparity between sophisticated and smaller firms.
Data, Model, and Strategy Implementation
Data Used
- Data Sources: 13F holdings, CRSP, Compustat, I/B/E/S, earnings call transcripts processed by ChatGPT
- Time Period: 2016–2023 (RAI spike post-2022)
- Asset Universe: US equities held by institutional investors
Model / Methodology
- Type of Model: Econometric panel regressions + difference-in-differences (DiD)
- Key Features:
- RAI = increase in R² from adding ChatGPT signals to standard financial models of holdings
- GPT answers 14 structured questions (firm earnings, capex, hiring, etc.) from call transcripts
- Outage-based DiD confirms causal link
Prompt: "Over the next quarter, how does the firm anticipate a change in:
- optimism about the US economy?
- optimism about the global economy?
- optimism about the financial prospects of their firm?
- optimism about the financial prospects of its industry?
- its earnings?
- its revenue?
- its wages and salaries expenses?
- demand for its products or services?
- production quantity of its products?
- prices for its products or services?
- prices for its inputs or commodities?
- its cost of capital or hurdle rate?
- its capital expenditure?
- its employment?"
Trading Strategy (If Applicable)
- Signal Generation: Use GPT-extracted firm-level forecasts to detect stock-level alpha
- Portfolio Construction: Tilt toward firms with favorable ChatGPT signals (e.g. strong earnings/guidance)
- Rebalancing Frequency: Quarterly (aligned with 13F disclosures and earnings calls)
- Enhancements: Filter signals by firm-policy dimensions (capex, employment)—where AI adds most value
Figure from the Paper
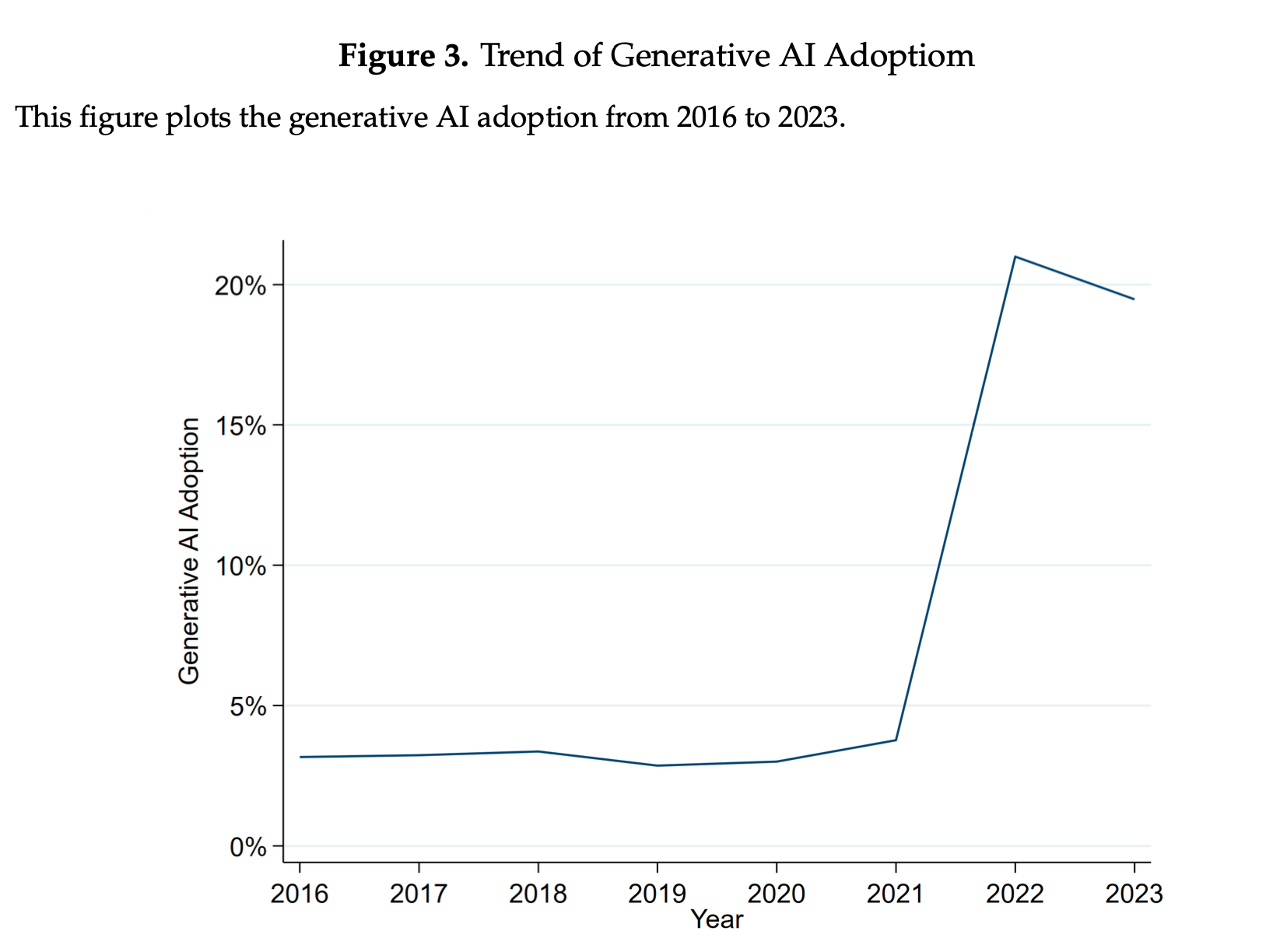
- Run Two Regressions per Fund-Quarter
Model 1 (Baseline):
\[ \text{HoldingsChange}_{i,j,t} = \gamma_i X_{j,t-1} + \varepsilon_{i,j,t} \]
Regress trades on traditional financial variables (e.g., size, ROA, analyst consensus).
Model 2 (With AI):
\[ \text{HoldingsChange}_{i,j,t} = \gamma_i X_{j,t-1} + \beta_i \text{GPT}_{j,t-1} + \varepsilon_{i,j,t} \]
Add ChatGPT signals to the model.
- Compute RAI Score
Define RAI as the increase in R² from adding GPT info:
\[ \text{RAI}_{i,t} = R^2_{\text{AI}, i,t} - R^2_{\text{Fundamental}, i,t} \]