Takeaway:
GPT-generated factors show exceptional return predictability and outperform traditional and data-mined factors, with many alphas unexplained by Fama-French models.
Key Idea: What Is This Paper About?
The paper explores using GPT-4 to autonomously generate novel stock return factors. Without using any financial data, GPT is prompted with basic variable definitions (price, volume, etc.), and outputs Python code and rationale for factor construction. These AI-crafted factors not only beat benchmarks but also offer robust economic explanations.
Economic Rationale: Why Should This Work?
GPT integrates financial theory, market intuition, and statistical logic. Its factors reflect investor behavior, market anomalies, and price/volume dynamics.
Relevant Economic Theories and Justifications:
- Behavioral Finance: GPT captures reversal/momentum linked to overreaction/underreaction.
- Liquidity Theory: Volume-based signals infer capital flow and market sentiment.
- Risk Premia: Many factors exploit volatility and trend-based risk-return tradeoffs.
Why It Matters:
Unlike data mining, GPT’s knowledge-based approach yields interpretable, economically grounded, and high-performing factors, reducing black-box risk.
How to Do It: Data, Model, and Strategy Implementation
Data Used
- Data Sources: CRSP (OHLCV US stocks)
- Time Period: Backtests from 2000–2022, primary testing Oct 2021–Dec 2022
- Asset Universe: US-listed equities
Model / Methodology
- Type of Model: GPT-4 LLM (no fine-tuning)
- Prompted Inputs: Definitions of financial variables (not raw data)
- Outputs: Factor formulas + Python code
- Selection: 35 fully functional novel factors
- Evaluation: Long-short and long-only portfolios, static and dynamic multi-factor strategies
- Benchmark Models: CAPM, Fama-French 3 and 5 factor regressions
Trading Strategy
- Signal Generation: Daily factor rankings based on GPT-created indicators
- Portfolio Construction:
- Long-short: Top 50% long, bottom 50% short
- Multi-factor: Averaging normalized factor signals
- Rebalancing Frequency: Daily
- Polarity Adjustment: Based on historical return sign (static) or cumulative return (dynamic)
Key Table or Figure from the Paper
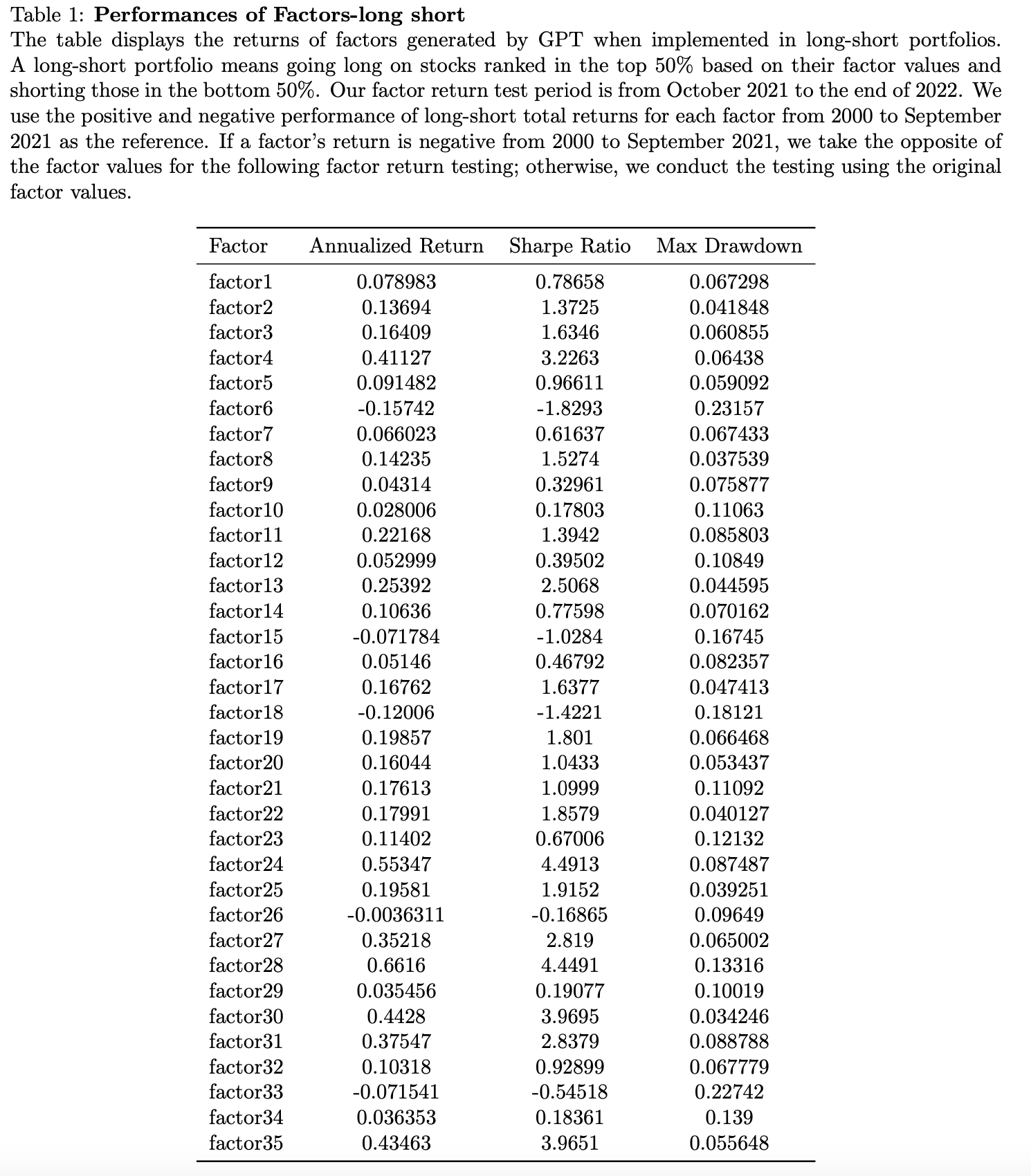
Explanation:
- Shows individual factor performance under long-short strategy.
- Best-performing factor (factor28) had 66.16% annualized return, Sharpe = 4.45, and low drawdown (13.3%).
- Over 30 of 35 GPT-generated factors yielded positive long-short returns.
- Alphas remained significant across both short-term and 20-year backtests, demonstrating robustness.
Final Thought
🚀 GPT-4 doesn’t just talk finance—it builds outperforming factors that rival human experts and revolutionize quant research.
Paper Details (For Further Reading)
- Title: GPT’s Idea of Stock Factors
- Authors: Yuhan Cheng, Ke Tang
- Publication Year: 2023
- Journal/Source: SSRN Working Paper
- Link: https://ssrn.com/abstract=4560216