Takeaway:
LLMs, particularly ensemble and Baichuan models, generate daily long-short signals from news that deliver extremely strong returns and alpha in the Chinese stock market.
Key Idea: What Is This Paper About?
This paper shows that large language models (LLMs) can extract predictive signals from Chinese news articles. These signals—news tone and return forecasts—lead to long-short portfolios with annualized returns between 35% and 88%. The ensemble model of 6 LLMs outperforms all others, demonstrating LLMs' potential in enhancing return prediction and market efficiency.
Economic Rationale: Why Should This Work?
LLMs can understand nuanced, high-dimensional text—something traditional NLP struggles with in Chinese. This allows them to identify fundamental signals missed by the market.
Relevant Economic Theories and Justifications:
- Limits to Arbitrage: Chinese markets have high retail participation and low institutional sophistication.
- Slow Information Diffusion: Prices incorporate LLM signals over 2+ days.
- Market Inefficiency: News with uncommon characters or from central media creates more friction.
Why It Matters:
This demonstrates how LLMs can extract alpha from public information in inefficient markets—bridging gaps left by both human analysts and simpler NLP tools.
Data, Model, and Strategy Implementation
Data Used (If Applicable)
- Data Sources: ChinaScope SmarTag (news), WIND & CSMAR (financial data)
- Time Period: 2008–2023 (train: 2008–2018, test: 2019–2023)
- Asset Universe: Chinese A-shares (2,193,371 news-stock pairs)
Model / Methodology (If Applicable)
- Type of Model: Pretrained LLMs (BERT, FinBERT, RoBERTa, Baichuan, ChatGLM, InternLM, Ensemble)
- Key Features: News tone (logistic model), return forecast (linear regression)
- Training Approach: Expanding window cross-validation, L2 regularization
- Evaluation: Fama-MacBeth regressions and CH4 four-factor alpha
Trading Strategy (If Applicable)
- Signal Generation: News tone and return forecast from LLM embeddings
- Portfolio Construction: Long top decile, short bottom decile (daily)
- Rebalancing Frequency: Daily (open-to-open)
Key Table or Figure from the Paper
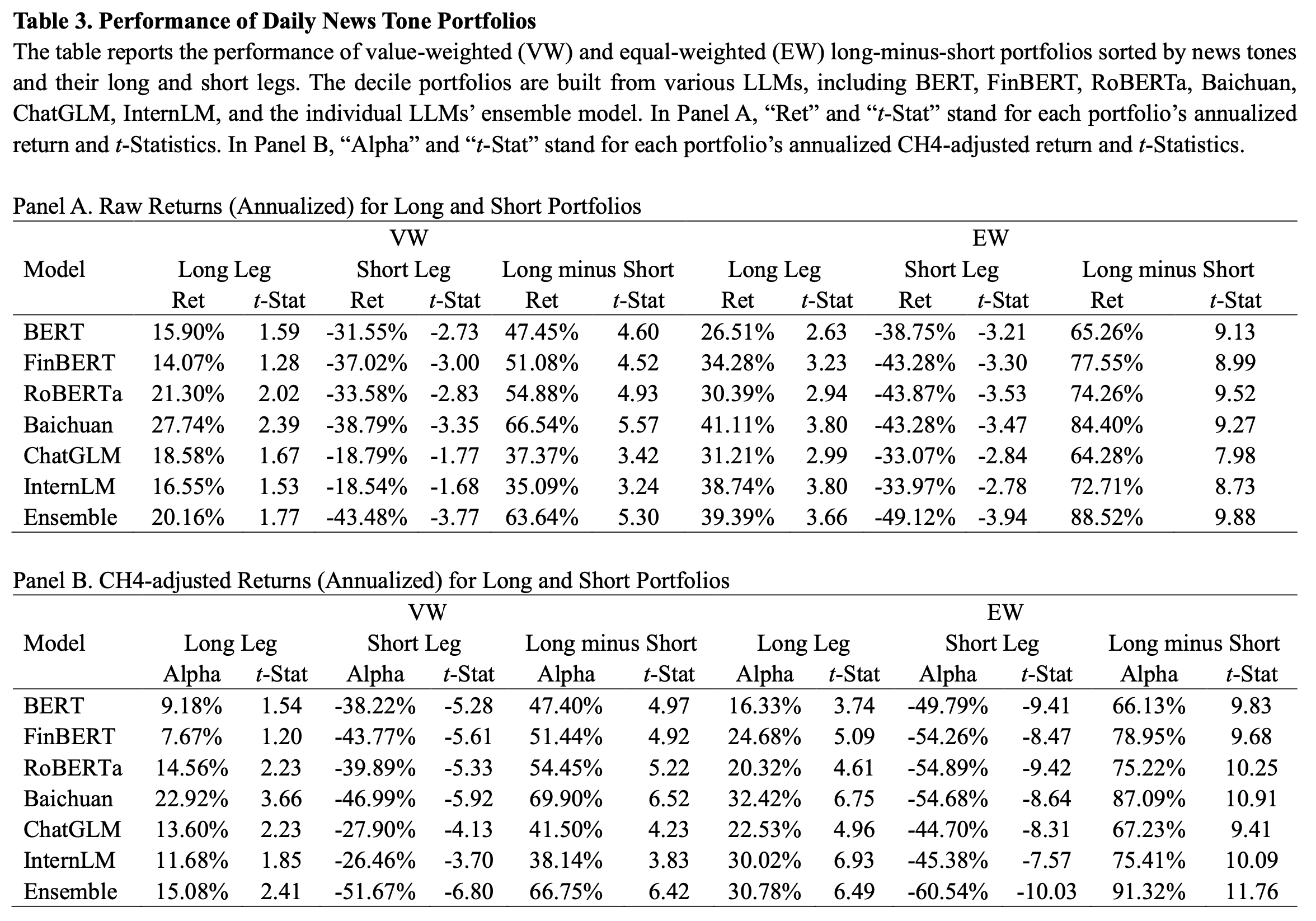
Explanation:
This table reports performance of long-short portfolios sorted by news tone. The ensemble model delivers an 88.5% EW return, 91.3% CH4-alpha, and t-stats > 11. This confirms that LLMs—especially ensembles—extract highly predictive signals from news.
Final Thought
🧠 LLMs can decode Chinese news into alpha—pushing the frontier of quant investing in emerging markets.
Paper Details (For Further Reading)
- Title: Large Language Models and Return Prediction in China
- Authors: Lin Tan, Huihang Wu, Xiaoyan Zhang
- Publication Year: 2024
- Journal/Source: SSRN Working Paper
- Link: https://papers.ssrn.com/sol3/papers.cfm?abstract_id=4712248