💡 Takeaway:
Market microstructure still matters. Features like VPIN and Amihud can forecast short-term market dynamics—even in the machine age.
Key Idea: What Is This Paper About?
This paper uses machine learning (random forests) to evaluate how well classic microstructure variables predict changes in price dynamics. The authors examine 87 liquid futures contracts and find that features like VPIN and Amihud illiquidity remain powerful predictors of volatility, spreads, and higher moments—even in modern, fast, algorithmic markets.
Economic Rationale: Why Should This Work?
📌 Relevant Economic Theories and Justifications:
- Asymmetric Information: Measures like VPIN detect toxic order flow.
- Liquidity Frictions: Amihud’s measure captures illiquidity-driven price impact.
- Execution Risk: Roll and Kyle measures capture transaction cost dynamics.
- Non-Normality & Serial Correlation: Higher moments matter in microstructure volatility models.
📌 Why It Matters:
These signals work out-of-sample across asset classes and horizons—suggesting market microstructure features still capture real economic frictions in algorithmic markets.
How to Do It: Data, Model, and Strategy Implementation
Data Used
- Markets: 87 global futures contracts (2012–2017)
- Frequency: Tick data aggregated into dollar-volume bars (~50 bars/day)
- Features:
- Roll Measure, Roll Impact
- Kyle’s Lambda
- Amihud Illiquidity
- VPIN
- VIX Futures
- Targets:
- Directional change in: bid-ask spread, volatility, Jarque-Bera, kurtosis, skewness, autocorrelation
Model / Methodology
- Classifier: Random Forest (100 trees, balanced sampling)
- Labels: Binary classification (increase vs. decrease)
- Accuracy Evaluation: 10-fold Purged Cross-Validation
- Feature Importance Measures:
- MDI (Mean Decreased Impurity, in-sample)
- MDA (Mean Decreased Accuracy, out-of-sample)
Trading Strategy (Derived Application)
- Signal Generation:
- Forecast short-term volatility increases (or non-Normality) using VPIN and Amihud
- Use Cases:
- Increase volume participation during rising volatility forecasts
- Risk-manage VaR models based on JB-stat, skew, or kurtosis forecasts
- Design spread-optimizing execution algorithms
- Model Execution:
- Use retrained ML models weekly using rolling 250-bar lookback
- Apply across futures, FX, or equity options markets
Key Table or Figure from the Paper
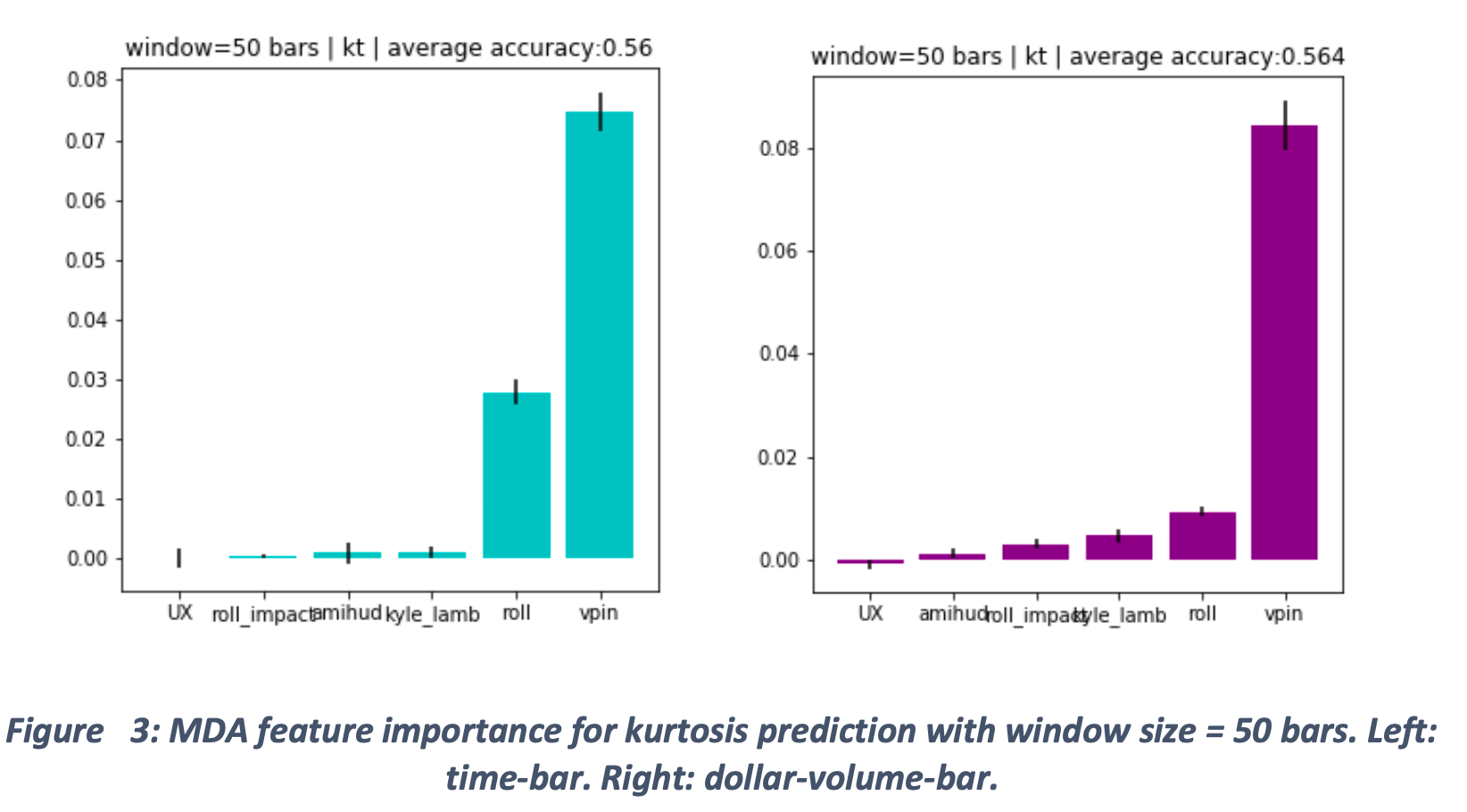
📊 Reference: [Figure 3] – Feature Importance for Kurtosis Prediction (MDA)
📌 Explanation:
- VPIN shows highest out-of-sample importance for predicting kurtosis direction.
- Amihud ranks highly for longer lookbacks; VIX underperforms.
- Confirms that VPIN (microstructure-informed measure) captures crash risk better than volatility proxies like VIX.
Final Thought
💡 Despite fast machines and big data, market frictions still shape the future—and microstructure measures still matter. 🚀
Paper Details (For Further Reading)
- Title: Microstructure in the Machine Age
- Authors: David Easley, Marcos López de Prado, Maureen O’Hara, Zhibai Zhang
- Publication Year: 2019
- Journal/Source: SSRN Working Paper
- Link: https://ssrn.com/abstract=3345183