Key Performance Metrics
📊 How Well Does This Strategy/Model Perform?
- Sharpe Ratio (Equal-Weighted L/S): 4.29
- Average Daily Return (L/S): 33 bps
- Risk-Adjusted Alpha (FF5+MOM): 4.3% per day (EW L/S)
- Outperformance vs RavenPack: SESTM earns 33 bps vs 18 bps/day
đź’ˇ Takeaway:
SESTM delivers strong predictive power and out-of-sample alpha. It outperforms both RavenPack and dictionary-based methods while using a transparent, white-box ML approach.
Key Idea: What Is This Paper About?
The paper introduces SESTM (Sentiment Extraction via Screening and Topic Modeling), a three-step supervised learning model to extract predictive sentiment from financial news. Instead of relying on pre-built dictionaries, SESTM learns which words matter for future returns and assigns them custom weights. It shows this model yields better predictions and more profitable trading strategies.
Economic Rationale: Why Should This Work?
📌 Relevant Economic Theories and Justifications:
- Limited Attention: Investors process news with delay, especially for small or volatile stocks.
- Textual Complexity & Novelty: Fresh news and complex sentiment signals are assimilated into prices more slowly.
- Information Frictions: Differences in speed of assimilation suggest inefficiencies that can be exploited.
📌 Why It Matters:
SESTM shows how tailored sentiment extraction reveals information traditional methods miss, enabling consistent alpha generation using public news.
How to Do It: Data, Model, and Strategy Implementation
Data Used
- News Source: Dow Jones Newswires (1989–2017)
- Price Data: CRSP, TAQ
- Training Sample: 1989–2012
- Out-of-Sample: 2004–2017
- Articles Processed: ~6.5 million single-stock articles
Model / Methodology
- Screening: Select sentiment-charged words using correlation with return direction.
- Topic Modeling: Assign word weights based on predictive ability using supervised topic modeling.
- Scoring: Aggregate to article-level sentiment with penalized likelihood.
- Key Tuning: Optimized using cross-validation on 5-year rolling windows.
- Portfolio Construction:
- Long top 50 sentiment stocks
- Short bottom 50
- Rebalanced daily, open-to-open returns
Trading Strategy (SESTM Long-Short)
- Weighting Schemes: Equal-weighted (preferred), and value-weighted
- Holding Period: 1 day (can be extended with decay-weighting)
- Transaction Costs: Handled via turnover-constrained variants (EWCT strategy)
Key Table or Figure from the Paper
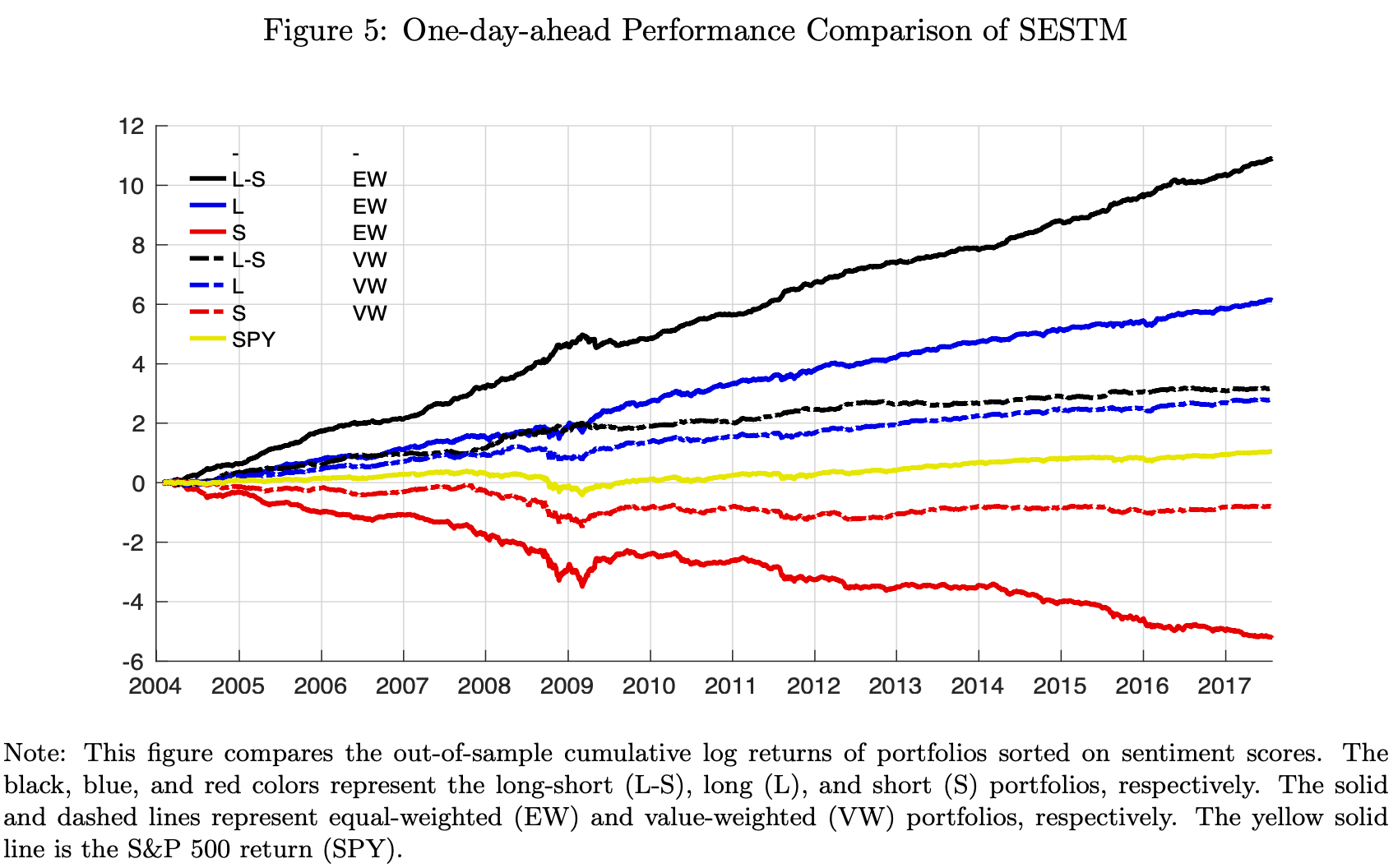
📌 Explanation:
- Shows performance of equal- and value-weighted long, short, and long-short portfolios using SESTM sentiment.
- The equal-weighted long-short portfolio consistently outperforms S&P 500 (SPY), including during crises.
- The strategy generates pure alpha: over 90% of return not explained by FF5+MOM factors.
Final Thought
💡 A machine that reads news and beats the market? SESTM proves it's possible—with transparency and theory to back it up. 🚀
Paper Details (For Further Reading)
- Title: Predicting Returns With Text Data
- Authors: Zheng Tracy Ke, Bryan T. Kelly, Dacheng Xiu
- Publication Year: 2019 (NBER Working Paper 26186)
- Journal/Source: NBER Working Paper
- Link: https://www.nber.org/papers/w26186