💡 Takeaway:
The Policy Change Index (PCI) signals policy shifts in China by measuring how surprising new propaganda is compared to past editorial patterns—offering early insight into structural economic turns.
Key Idea: What Is This Paper About?
The paper constructs a real-time indicator—the Policy Change Index (PCI)—using machine learning to detect changes in the editorial priority of China’s state newspaper, the People’s Daily. By training a model to predict whether an article should appear on the front page and tracking how its performance changes over time, the PCI captures editorial surprises that precede major policy changes. The index successfully leads key moments in Chinese history and offers ongoing macro predictive power.
Economic Rationale: Why Should This Work?
📌 Relevant Economic Theories and Justifications:
- Propaganda as Policy Signaling: In authoritarian regimes, media forecasts policy—not just reflects it.
- Editorial Placement as Priority Proxy: Front-page articles in People’s Daily represent regime goals.
- Predictive Surprises: Large “surprises” in model classification performance indicate a break in political messaging, often preceding reforms.
- No Labeling Required: Model performance deterioration itself becomes the signal—no need to manually label policy content.
📌 Why It Matters:
This “language-free” approach bypasses NLP complexity and taps directly into the CCP’s internal policy coordination system—making it a powerful tool for forecasting Chinese policy risk.
How to Do It: Data, Model, and Strategy Implementation
Data Used
- Source: Full text of People’s Daily (1946–2019)
- Articles: 1.9 million (226k front-page)
- Metadata: Publication date, article length, page number, weekday/weekend flags
Model / Methodology
- Model Task: Predict front-page placement using text + metadata
- ML Architecture:
- Word embedding (People’s Daily-trained)
- Recurrent neural network (GRU)
- Multilayer perceptrons
- Policy Signal (PCI):
- PCI = |F1(Training) − F1(Forecast)|
- Surprise jump in F1 score → high likelihood of near-term policy change
- Update Frequency: Quarterly with a five-year rolling training window
Trading Strategy (Macro Timing / China Risk Exposure)
- Signal:
- Spike in PCI indicates a major policy change in the next quarter
- Analyze article types (false negatives or false positives) to infer substance
- Execution Ideas:
- Increase/decrease exposure to China-sensitive assets (e.g., H-shares, commodities, EM FX)
- Adjust tilt toward SOEs or private sector based on inferred policy lean
- Risk Management:
- Combine with macro/micro filters to reduce noise
- Avoid relying on PCI for covert or crisis-driven moves (e.g., Tiananmen 1989, 2008 stimulus)
Key Table or Figure from the Paper
📊 Reference: [Figure 1] – Policy Change Index and Major Policy Events in China
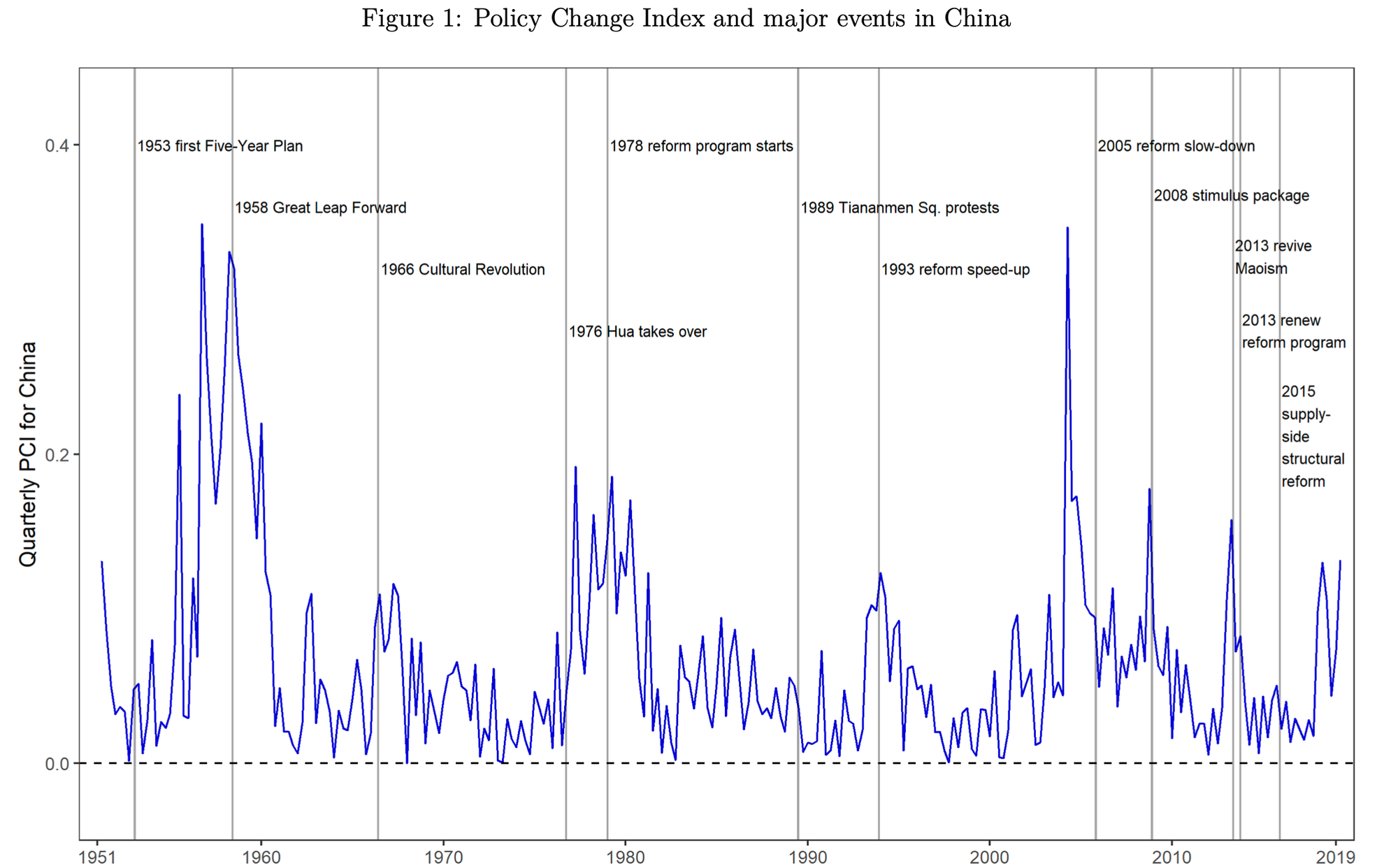
📌 Explanation:
- Plots PCI over time with vertical bars marking key historical reforms
- Spikes in the PCI precede most major events:
- 1958: Great Leap Forward
- 1978: Economic reform program
- 1993: Reform speed-up (Socialist Market Economy)
- 2004: Reform slowdown (Harmonious Society)
- 2018–19: Xi era shifts
- Validates PCI as a true leading indicator of structural change in China
Final Thought
💡 Want to know China’s next move? Watch the front page, not just the headlines. 📰🚀
Paper Details (For Further Reading)
- Title: Reading China: Predicting Policy Change with Machine Learning
- Authors: Julian TszKin Chan, Weifeng Zhong
- Publication Year: 2019
- Journal/Source: Working Paper
- Link: https://policychangeindex.org/pdf/Reading_China.pdf