📈 Performance
- Image-based CNN models generate out-of-sample Sharpe ratios up to 7.2 (equal-weight, weekly).
- Outperforms traditional trend-following strategies like momentum and short-term reversal.
- Profits persist across different time horizons (weekly, monthly, and quarterly).
💡 Key Idea
The authors rethink trend-based predictability by leveraging convolutional neural networks (CNNs) to analyze price charts as images rather than relying on predefined patterns like momentum or reversal. This data-driven approach automatically extracts return-predictive price patterns.
📚 Economic Rationale
Traditional technical analysis relies on human-recognized patterns. This study automates pattern recognition using deep learning, exploiting context-independent price trends that work across time scales and markets. The findings align with behavioral finance theories that suggest prices exhibit persistent, complex patterns.
🚀 Practical Applications
- Enhancing quantitative trading models by incorporating AI-driven price trend signals.
- Developing systematic strategies that adjust dynamically to market conditions.
- Applying transfer learning: models trained on U.S. stocks perform well in international markets and at different trading frequencies.
🔧 How to Do It
Data Used:
- Daily U.S. stock market data (1993–2019) from CRSP.
- Price charts generated from Open-High-Low-Close (OHLC) and volume data over 5, 20, and 60 days.
Model & Methodology:
- CNN Model: Uses deep learning to extract predictive price patterns from stock charts.
- Training Data: Historical stock price images labeled by future return direction.
- Prediction Task: CNN estimates probability of positive returns over different horizons (5, 20, 60 days).
- Portfolio Construction: Stocks are sorted into deciles based on CNN forecasts, forming long-short portfolios.
Strategy Execution:
- Convert price and volume data into images.
- Train CNN to recognize predictive patterns.
- Sort stocks into deciles based on CNN's probability estimates.
- Construct long-short portfolios (buy top decile, short bottom decile).
- Rebalance weekly, monthly, or quarterly.
📊 Key Figure:
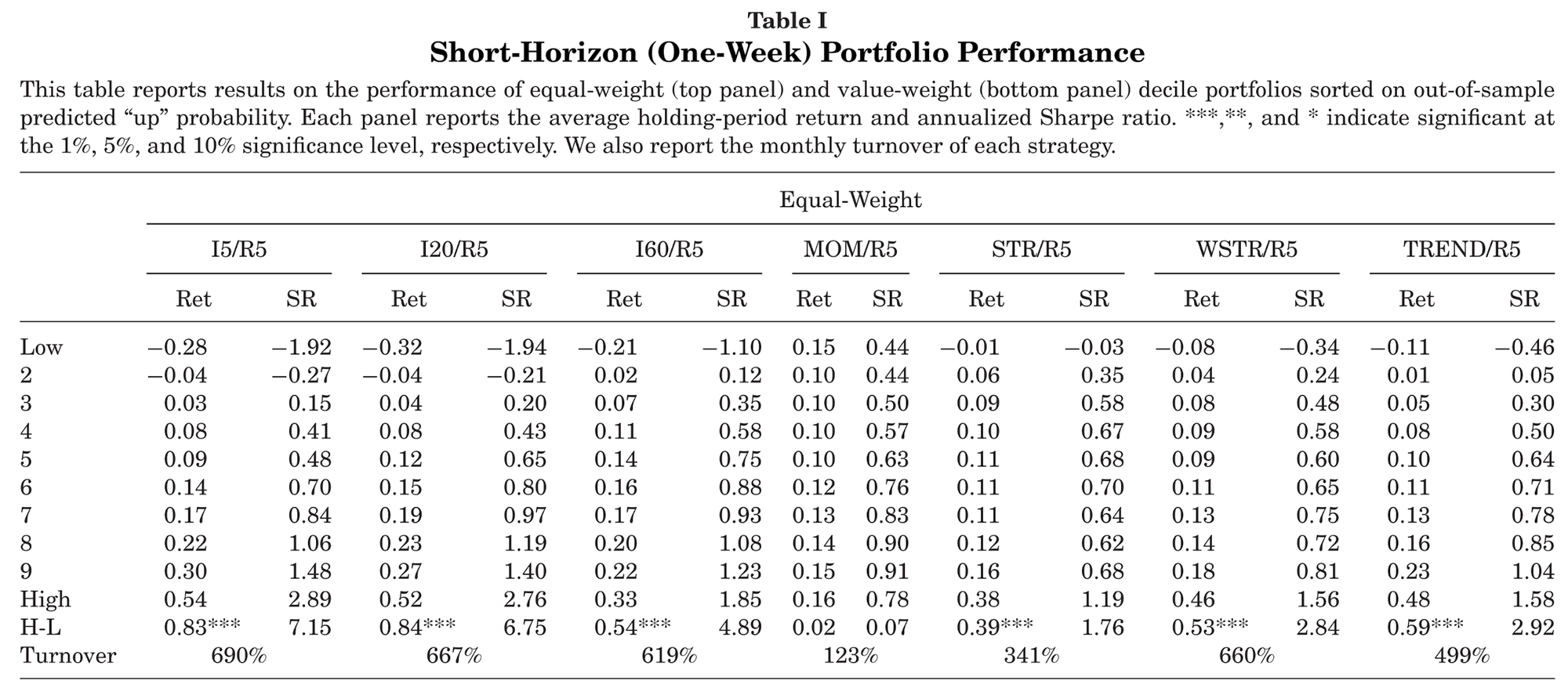
- CNN-based long-short portfolios deliver higher Sharpe ratios than momentum and reversal strategies.
- Equal-weighted strategy Sharpe ratio reaches 7.2, compared to 2.9 for momentum and reversal.
- Value-weighted portfolios also show strong performance (Sharpe ratio ~1.7).
📊 Sharpe Ratios
Period | CNN (EQ) | CNN (VW) | MOM | STR | WSTR | TREND |
---|---|---|---|---|---|---|
Weekly | 7.2 | 1.7 | 0.1 | 1.8 | 2.8 | 2.9 |
Monthly | 2.4 | 1.4 | 0.7 | 1.2 | 1.2 | - |
Quarterly | 1.3 | 1.3 | 0.1 | 0.7 | 0.7 | - |
- CNN-based strategies dominate traditional price trend strategies in weekly trading, achieving a Sharpe ratio of 7.2 (equal-weighted).
- CNN models maintain strong performance at monthly (2.4) and quarterly (1.3) horizons, though weaker than short-term trading.
- Value-weighted portfolios (more relevant for institutional investors) still deliver Sharpe ratios above 1.0.
- Momentum and reversal strategies underperform significantly compared to deep learning models.
📄 Paper Details
Authors: Jingwen Jiang (University of Chicago), Bryan Kelly (Yale, AQR), Dacheng Xiu (University of Chicago)
Published in: Journal of Finance, December 2023
DOI: 10.1111/jofi.13268